AlphaFold uses these signals as the basis for its predic- We also use nuclear magnetic resonance (NMR) for struc- tions, however, good predictions are not possible if such tural elucidation — particularly for small proteins in solu- signals are only sparingly available. AlphaFold therefore tion and to investigate interactions and their dynamics has its limitations — for example, when predicting in the cell. Using NMR with large proteins is di昀케cult and structures of viral proteins, antibody-antigen complex- time-consuming, however, and the technique relies on es, or when analyzing the in昀氀uence of mutations on a isotope marking. On the other hand, NMR is highly e昀昀ec- structure. There are also limitations when it comes to tive for rapidly “testing” folds or for speci昀椀cally “reading” investigating the binding of biological or synthetic mol- certain properties. ecules, such as when searching for new medications. Each of these methods has speci昀椀c advantages The con昀椀dence values provided by AlphaFold and disadvantages. The choice of method depends on usually re昀氀ect these uncertainties, enabling us to focus on the type of protein, the availability of resources and the the proteins or parts of proteins for which the prediction desired level of detail of the structure. is reliable and to show the cases where good predictions are not possible. SNI INSight: Are these methods now being replaced by AlphaFold? SNI INSight: What methods exist for experimental structural elucidation? Janani Durairaj: Experimental methods and AlphaFold complement one another. Timm Maier: Recent years have also brought huge ad- One useful analogy here is that of Wikipedia vances in experimental methods of structural elucidation. vs. ChatGPT. Carefully veri昀椀ed experimental structures X-ray crystallography continues to play a key role and is would correspond to Wikipedia in this analogy, while the ideally suited to delivering high-resolution structures of predictions made with AlphaFold correspond to ChatGPT. small protein molecules with a high throughput. With The veri昀椀ed experimental structures contain copious in- the Swiss Light Source at the Paul Scherrer Institute, for formation on speci昀椀c biologically relevant interactions example, we can analyze around a hundred proteins in and mechanisms for a smaller subset of known proteins. the space of a week — provided we have crystals to hand. AlphaFold2, on the other hand, delivers predictions for a X-ray crystallography is also ideal for fascinating special far greater number of proteins for which no experimental applications such as the analysis of metal ions. With the structures yet exist providing very good starting points SwissFEL X-ray free-electron laser, it is even possible to for further detailed study. track dynamic processes such as the binding of molecules in real time. Timm Maier: On the contrary, AlphaFold and related Larger proteins are a job for cryo-electron mi- methods represent a key contribution to the necessary fur- croscopy (cryo-EM), which now allows us to image even ther development of experimental analysis. Some 20–30 very large protein complexes and thereby to gain precise years ago, experimental structural determination focused information about their structure. On the downside, cryo- on elucidating new fundamental protein folds. In those EM is nowhere near as fast as X-ray crystallography and days, the aim was to take data from genome research and generates incredibly large volumes of data that we have use it to explore the fold space of proteins. This process to save for a long time — which represents an enormous has long since been completed. Today, experimental struc- cost factor. tural biologists are addressing how dynamic interactions and various functional states of proteins help make cellu- lar life possible — ideally by considering proteins in their natural environment within the cell. Here, AI-generated models of the folding of individual proteins are a useful tool when it comes to recognizing certain proteins in a cell. At the same time, machine learning methods also allow researchers to design new proteins on the computer that perform very speci昀椀c tasks — such as breaking down a speci昀椀c plastic. We recently demonstrated for the 昀椀rst time in a block course how well this protein design pro- cess now works and how quickly we can arrive at astonish- ing results and completely novel proteins. Experimental structural elucidation is then needed in order to optimize the protein design and improve the design methods. In Timm Maier and his team combine experimental and computer-assisted this sense, machine learning actually creates additional methods such as AlphaFold to study isolated proteins and proteins in demand for experimental structural elucidation. cells in order to gain a better understanding of the dynamics and function in the cell. SNI INSight December 2024 7
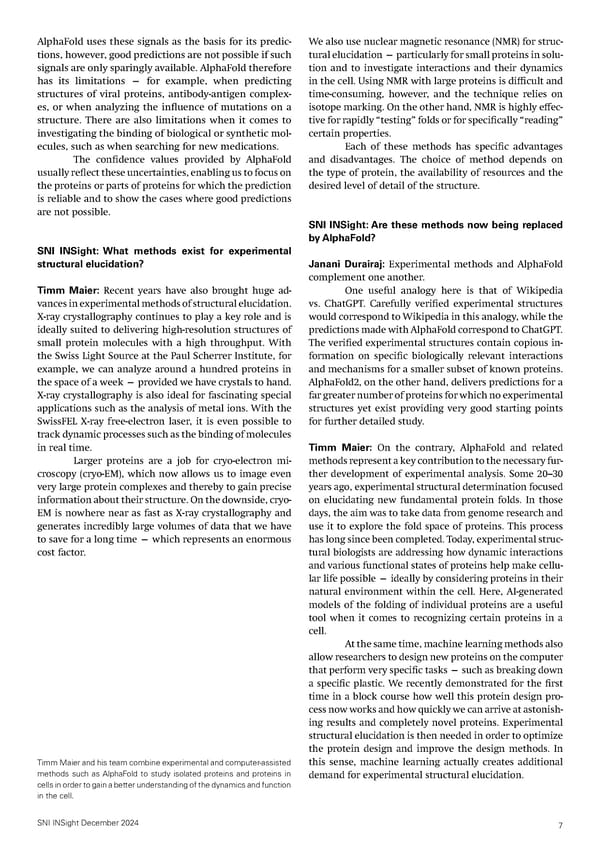